
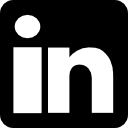
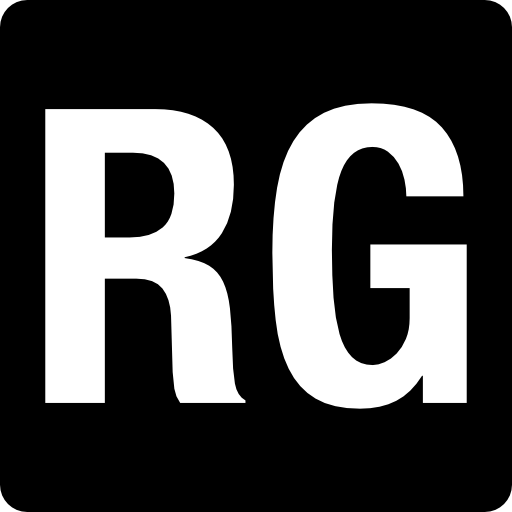
I am a Ph.D. candidate in the Department of Computer Science and Engineering at Michigan State University (MSU), skilled in in wireless communications and networking, signal processing, and machine learning. My research interests include design, simulation, and analysis of networking protocols for wireless networks.
at Michigan State University
at University of Louisville
at University of Tehran
at Iran University of Science and Technology
Full List of Publications →
DeepMux is deep-learning-based MU-MIMO-OFDMA transmission scheme for 802.11ax networks. DeepMux comprises DLCS and DLRA. DLCS uses DNNs to reduce the airtime overhead of 802.11 protocols and DLRA employs a DNN to solve the mixed integer resource allocation problem.
UD-MIMO enables concurrent uplink transmission in the absence of fine-grained synchronization. Its enabling technique is a scheme to decoding uplink packets from asynchronous users. UD-MIMO makes it possible for WLANs to significantly improve their uplink throughput without requiring synchronization.
We proposed a spectrum sharing scheme for cognitive radio networks where secondary users have no knowledge about the primary network. We have built a prototype of our scheme that showed the secondary network could coexist with commercial Wi-Fi and LTE devices without degrading their performance.
LB-SciFi is a learning-based feedback framework for MU-MIMO in WLANs. It employs DNN autoencoders to compress CSI in WiFi networks, thereby conserving airtime and improving spectral efficiency. Experimental results show that it offers 73% airtime overhead reduction and 69% throughput gain.
We proposed a downlink NOMA scheme for WLANs including precoder design, user grouping, and successive interference cancellation (SIC). We prototyped the proposed scheme and experimental results show that the proposed downlink NOMA significantly improves the weak user’s date rate and sum rate.
TCCI is an interference management scheme enabling concurrent transmission in WLANs. TCCI requires neither network-wide synchronization nor inter-network data sharing, and therefore is amenable to implementation. We have illustrated TCCI compatibility with commercial devices.
This paper proposes a practical underlay spectrum sharing scheme for CRNS where the primary users are oblivious to secondary users.
The key components of our scheme are two MIMO-based interference cancellation techniques, blind beamforming and blind interference cancellation techniques.
In this paper, we propose an energy-efficient IoT communication scheme by taking advantage of the existing WiFi infrastructure.
EE-IoT will not only avoid monthly service charge for the end users but also maintain a low power consumption for IoT devices.
In this project, we aim to increase the convergence of federated learning in a deadline-constrained networks such as VANETs, where local models need to be polled considering vehicles deadines.
In most federated learning frameworks, the non-selected users store data samples. This paradigm is not suited for low-end storage-limited users. In this project, we aim to revisit this paradigm.
If you need real experimental datasets to evaluate your ideas, you can use the following datasets. No permission is required.
This test measures the channel gain difference for 3600 users pairs to investigate the statistics of the gain difference among all possible user pairs for using in NOMA. . We have measured the average channel gain of users on 2.485 GHz with 5MHz bandwidth.
This test aims at measuring the channel frequency response of an indoor wireless
environment over 25 MHz bandwidth in 2.1 GHz band.
Based on the measured results, we can study the rank deficiency of MIMO channels to have a better DoF allocation for MIMO transmissions.
This test examines the channel reciprocity for USRP N210 at 2.4 GHz with 5MSps for 7 hours. Wi-Fi legacy frame is used to measure the uplink and downlink channels over 52 valid subcarriers. We used Argos as a relative calibration method to measure and compensate the mismatch for 4 N210 USRPs with SBX daughterboards.